Machine Learning for Climate Science
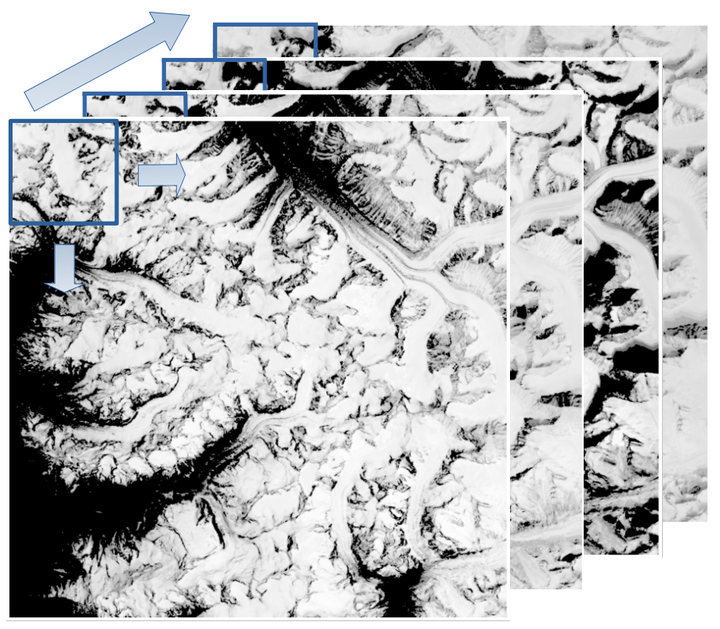
Atmospheric processes are highly non-linear and chaotic. However, recurring patterns in the climate system lead to predictable tendencies in weather or long-term climate change impacts. Machine Learning is ideally suited to identify these subtle patterns in complex data and exploit them to generate predictions. We aim to develop machine learning models that can generate skilful predictions at time scales that are difficult to model with numerical methods (e.g., sub-seasonal to seasonal), or for processes which are complex to model numerically (e.g., glacier movement prediction).
Main Research Interests:
- Machine Learning for Sub-seasonal to Seasonal Forecasting
- Glacier Movement Prediction using Satellite Data